Hi, I'm Ben Koska
I am an AI researcher passionate about advancing deep learning, currently based in Slovakia.
About me
What are your main research interests?
I focus on advancing synthetic data, exploring neural networks, and developing small language models for on-edge computing.
What do you enjoy beyond research?
I love diving into aerospace engineering concepts, tinkering with simulations, and brainstorming how AI can redefine the future of flight and space exploration.
Career
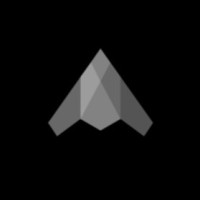
Stealth Deep Tech Startup
San Francisco, USA
I founded a stealth Deep Tech startup working on the future of Intelligence.
KOSKA GmbH
Vienna, Austria
I headed the software engineering department at KOSKA, as well as several artifical intelligence research projects.
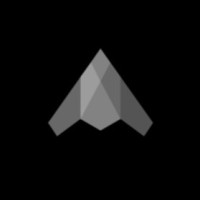
Stealth Compiler Startup
London, England
I co-founded a stealth Tech startup and worked as CEO there, while I oversaw the development of the product.
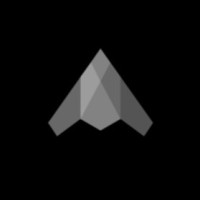
Stealth FinTech Startup
London, England
I co-founded a stealth FinTech startup and worked as CTO there, while I was working there I oversaw the development of the product and hiring of software engineers.
Scribbly
Boston, Massachusetts, United States
I worked as a Software Engineer focused on developing a Machine Learning-based news analysis system for a security platform, an incident reporting tool, and a web app for electronic business cards.
Publications
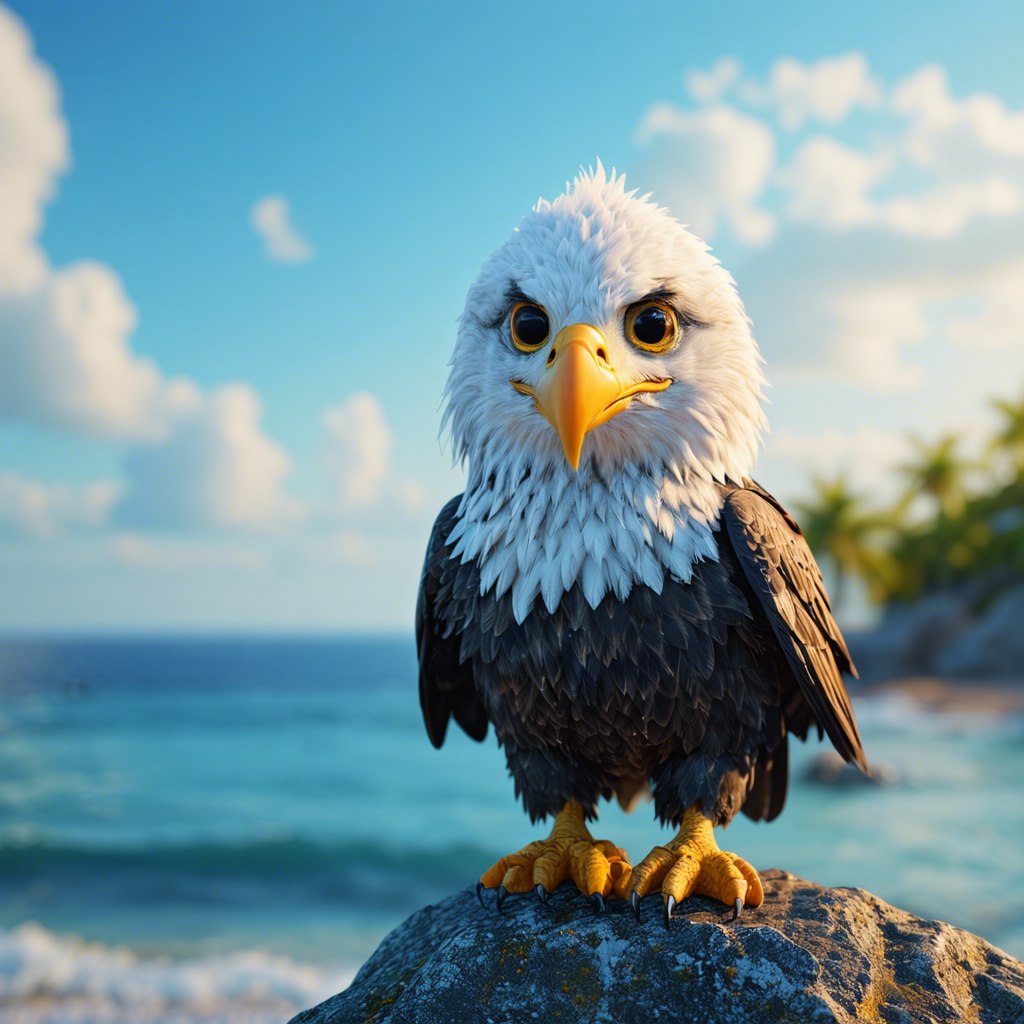
Towards Multi-Modal Mastery: A 4.5B Parameter Truly Multi-Modal Small Language Model
2nd International Conference On Foundation And Large Language Models
We present a novel 4.5B parameter small language model that can handle multiple input and output modalities, including text, images, videos, and audio. Despite its small size, the model achieves near state-of-the-art performance on a variety of tasks, demonstrating the potential of multi-modal models to tackle complex real-world problems. Our approach leverages recent advancements in language modeling and multi-task learning to create a versatile and high-performing model that can even be deployed for edge inference. Experimental results show the model's strong performance across multiple benchmarks, paving the way for further progress in multi-modal artificial intelligence.
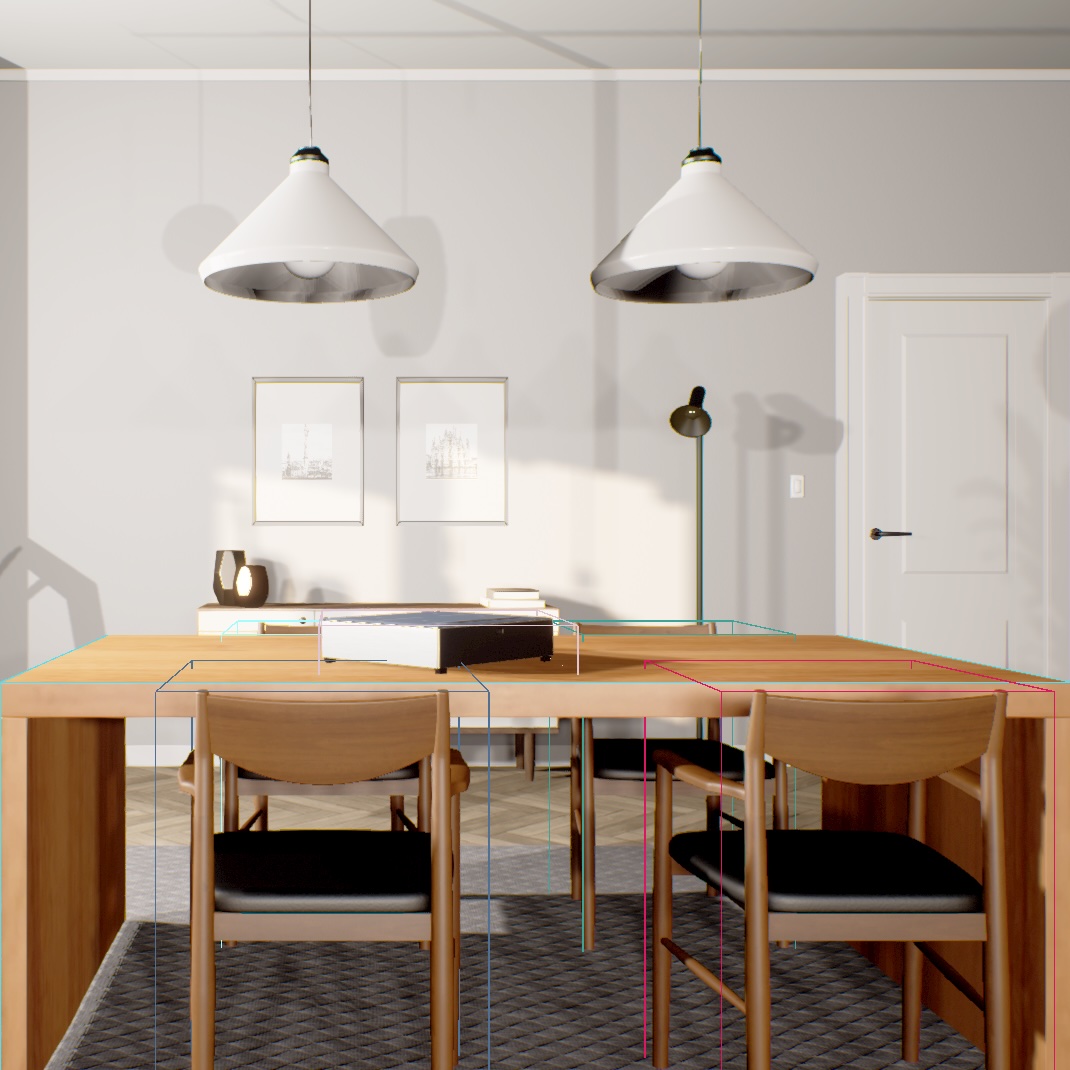
Simulated Realities are all you need: Synthetic Data for Enhanced Depth, Localization, and Object Detection in egocentric views
Under review
Augmented reality (AR) applications require precise spatial understanding to facilitate accurate mapping, object detection, and scene comprehension. However, real-world data for training such tasks is often difficult to obtain - and especially difficult to label - at scale and lacks the diversity needed for robust model performance. This paper introduces a synthetic data framework to advance AR capabilities, leveraging simulated environments with realistic lighting, textures, and complex scene interactions.